The Problem
- Create an Outfit Recommender System: Develop a system that provides outfit recommendations that are consistent in style yet rich in diversity. This means the system should suggest outfits that fit well within established style categories while also introducing variety to keep the user’s fashion choices fresh and inspired. The recommendations should be both practical and creative, catering to different tastes and preferences.
- Scalable MVP: Ensure the solution is a Minimum Viable Product (MVP). This MVP should be designed for fast deployment, allowing for quick market entry and early user feedback. At the same time, it must be scalable, and capable of handling increasing numbers of users and data without compromising performance or speed. The architecture should be flexible to support future enhancements and integrations.
- AWS Integration: Implement the solution on AWS to leverage its robust, scalable infrastructure. The system should seamlessly communicate with the mobile app, ensuring real-time updates and interactions. AWS services will be used to handle data storage, processing, and machine learning model deployment, providing a reliable backbone for the recommendation system.
The Challenge
Fashion is highly subjective, making it difficult to develop a system that can universally recommend stylish and diverse outfits. Individual preferences, sartorial literacy, and personal experiences greatly influence how people perceive and categorize styles. Developing an algorithm that can navigate these nuances and deliver personalized, accurate recommendations is a complex task.
The Solution
Feature Extraction with FashionFormer: Implementation of the FashionFormer model, capable of identifying 46 garment types and 294 distinct attributes, providing a robust foundation for feature extraction.
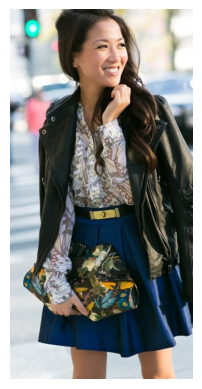
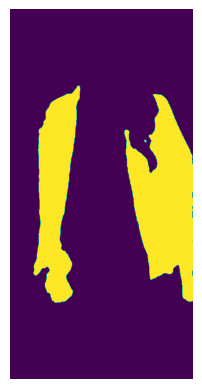
Style Clustering with Polylingual Topic Modeling: Used Polylingual Topic Modeling to categorize extracted attributes by body parts and applied Latent Dirichlet Allocation (LDA) to identify coherent style clusters. Employed an iterative process to refine style categorization, enhancing both coherence and diversity.
Similarity Search with FAISS: Implementation of Facebook AI Similarity Search (FAISS) to efficiently find stylistically similar outfits, handling large datasets accurately.
AWS Implementation:
- Image Processing: When an image is uploaded, it is processed by FashionFormer running on an EC2 instance.
- Data Storage: The resulting attributes and garments are stored in AWS RDS, while images are backed up in an AWS S3 bucket.
- Similarity Searches: Incoming similarity searches are processed on AWS EC2 instances.
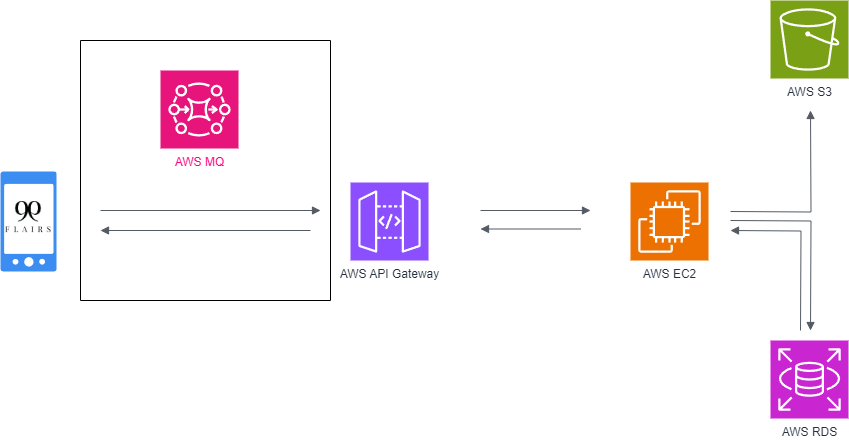
The architecture ensures that data flows seamlessly from user interactions on the app, through the message broker and API gateway, to the processing units and storage services. This design supports scalability, robustness, and efficient data management, ensuring that 99flairs can provide personalized and accurate outfit recommendations in real-time.
The Results
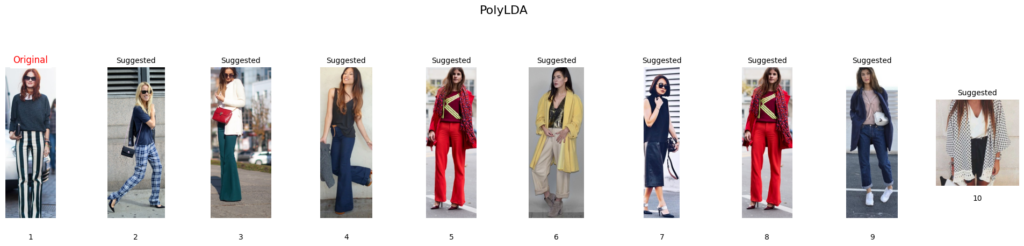
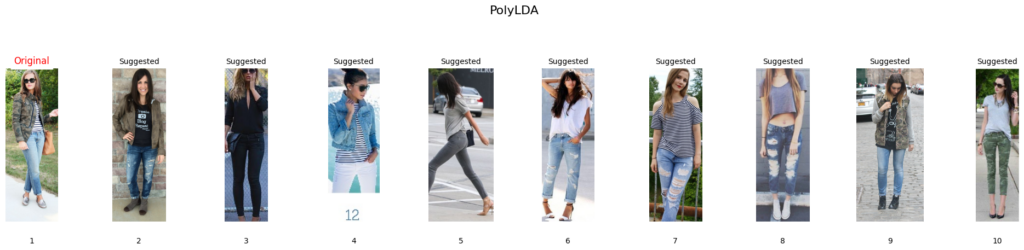
By navigating the complexities of style subjectivity and leveraging advanced machine learning techniques, this project successfully developed a sophisticated outfit recommendation system. This system not only addresses the core challenges of style prediction but also offers a practical solution that can inspire innovation in the fashion industry. For a detailed guide on the data science part with all my thought processes, code examples, and a detailed investigation of the results check out my Medium article. After language models reached a decent level in describing images this year (2024), I accepted the challenge to rebuild the system as a showcase using state-of-the-art Generative AI. You can read about the new implementation here or directly try out the new model by uploading an image here.